"AI sucks", Quantifying EMR burden, and Loneliness
Get Out-Of-Pocket in your email
Looking to hire the best talent in healthcare? Check out the OOP Talent Collective - where vetted candidates are looking for their next gig. Learn more here or check it out yourself.
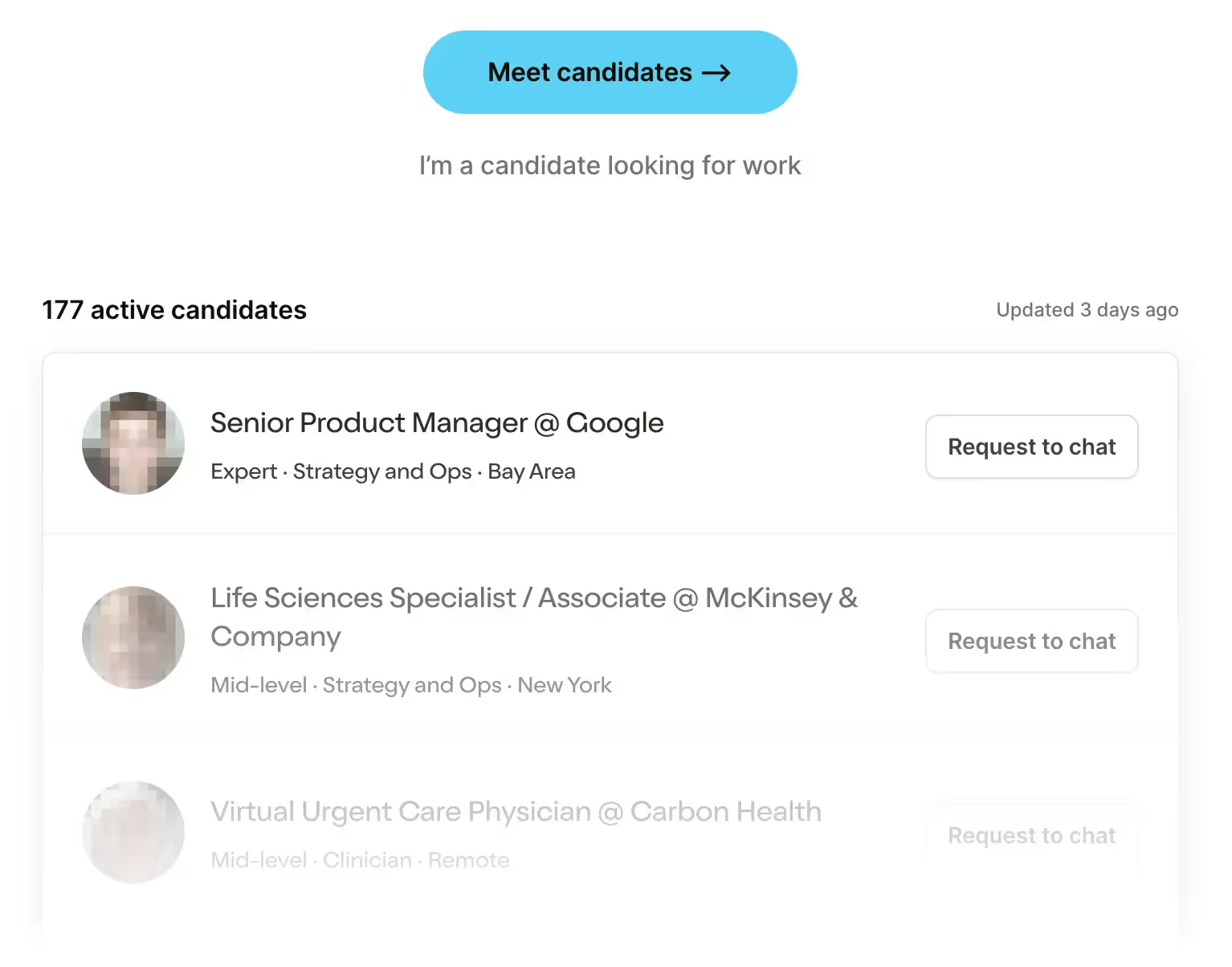
Healthcare 101 Crash Course
%20(1).gif)
Featured Jobs
Finance Associate - Spark Advisors
- Spark Advisors helps seniors enroll in Medicare and understand their benefits by monitoring coverage, figuring out the right benefits, and deal with insurance issues. They're hiring a finance associate.
- firsthand is building technology and services to dramatically change the lives of those with serious mental illness who have fallen through the gaps in the safety net. They are hiring a data engineer to build first of its kind infrastructure to empower their peer-led care team.
- J2 Health brings together best in class data and purpose built software to enable healthcare organizations to optimize provider network performance. They're hiring a data scientist.
Looking for a job in health tech? Check out the other awesome healthcare jobs on the job board + give your preferences to get alerted to new postings.
Hello. Here are some papers I liked.
Are you a robot?
We begin with another AI paper with an AI pun. I assume this is a requirement to be published at this point.

This paper sought to answer a few questions.
- If you give a doctor a recommendation, does the source of that recommendation change how they perceive the recommendation and choose to listen to it? In this case it compared suggestions coming from “AI” vs. “an expert radiologist” to see how the doctors react.
- Does the impact of #1 change depending on whether the physician receiving the advice is already an expert or not?
- How can we make healthcare sales people jealous of our email conversion rates
During recruitment, we sent emails to residency directors/coordinators at most institutes in the US and Canada that had residency programs in radiology (183 institutions), IM (479) and EM (238), and asked directors/coordinators to forward the email to residents and staff in that field. In addition, when we found physician emails available on the institution's website, we sent recruitment emails to them directly. This process led to approximately 1850 emails sent, resulting in 425 people opening the link to the Qualtrics survey page. 361 people then met our inclusion criteria, consented to participate, and started to look at cases. Finally, 265 people finished looking at cases and answered all of the post-survey questions about demographics, attitudes, and professional identity/autonomy - these are our final participants included in the analysis. This is about a 14.3% response rate given our initial 1850 recruitment emails.

What I like about the paper is the simplicity of the experiment’s design. Take two groups of physicians - specialists (radiologists) and generalists (internal medicine/emergency medicine). Give them 8 cases with images and advice about what the diagnosis is. For some people the advice is labeled as coming from an expert AI and for other people the advice is labeled as coming from “Dr. S. Johnson, an experienced radiologist”. The advice was actually generated by the researchers/some experts they consulted with. However, 2 out of every 8 cases have intentionally incorrect advice.

So the physicians get the cases and the advice, give a confidence score of how good the quality of the advice they got was, and then make a final diagnosis themselves.
The results are fun.
- The most important takeaway is that when given inaccurate advice, physicians will anchor to it regardless of whether they’re a specialist or generalist. This has important ramifications when we think about clinical decision support tools - the suggestions we present will have a high degree of influence on the decision the physician makes.
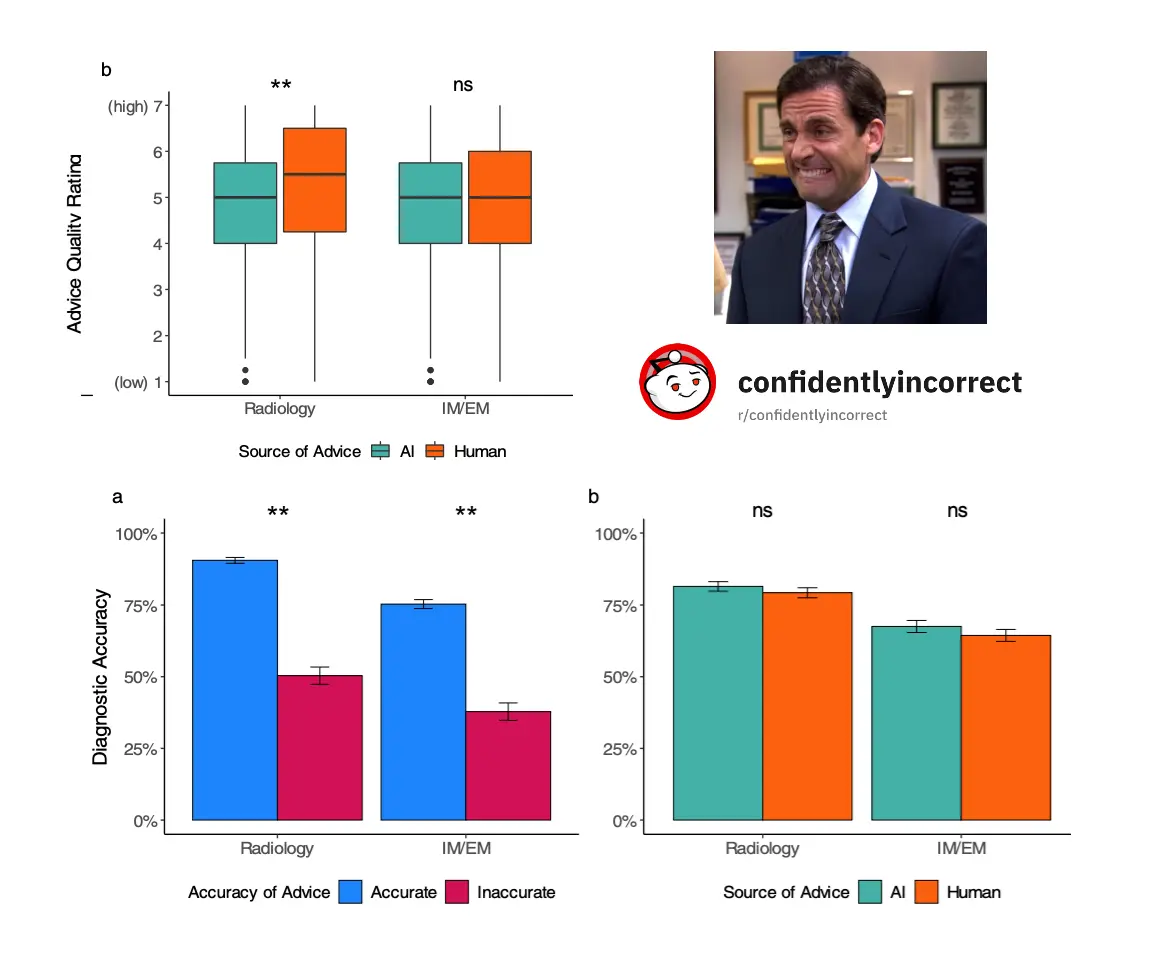
- Radiologists said AI gives bad advice relative to their esteemed fake colleague. This is revenge after being told for decades that their job is becoming obsolete because of AI and also working with less than stellar tools in the past. However, radiologists performed equally well regardless of who they thought was giving the advice, including messing up the cases where they were given inaccurate information. So even though they’re skeptical of the “AI” advice, they…still listen to it?
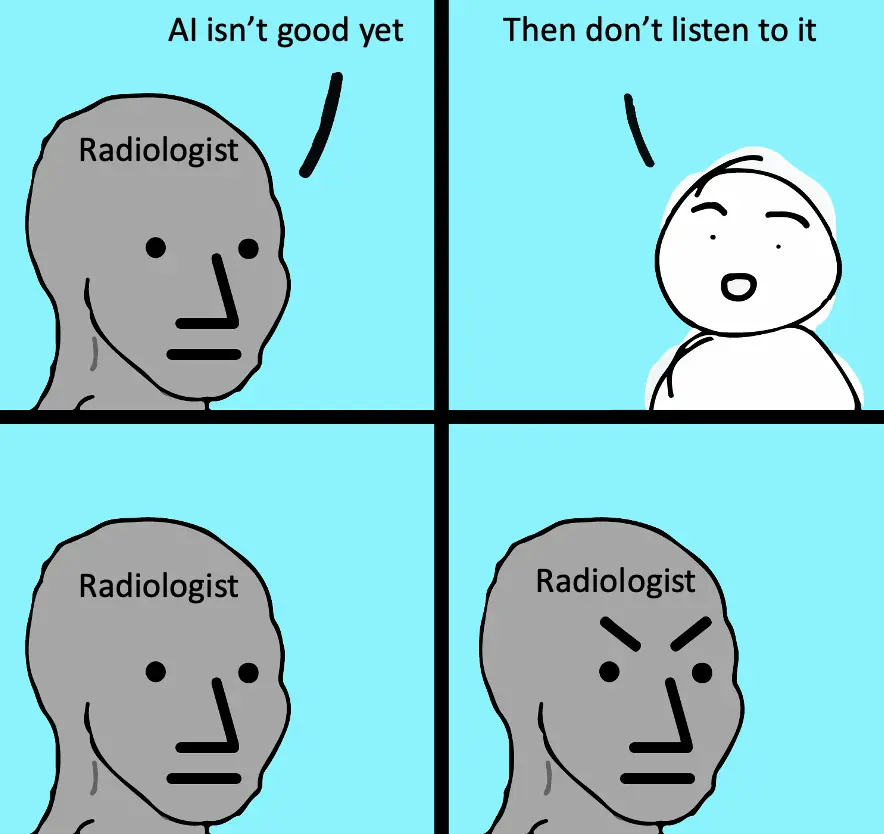
- There’s a lot of variance at an individual level. The graph is a reminder that there’s a wide range in physician diagnostic accuracy. IMO why we should have physician-specific centers of excellence programs.
- Radiologists are better performers (13.04% had perfect accuracy, 2.90% had ≤ 50% accuracy), than IM/EM physicians (3.94% had perfect accuracy, 27.56% had ≤ 50% accuracy). This makes sense since it’s the radiologist’s field of specialization.
- Physicians were considered “critical” if they managed to detect both wrong cases and ignore the advice, while they were “susceptible” if they got both cases wrong when given bad advice. 28% of radiologists were critical vs. 17% of generalists. ~28% of radiologists were susceptible vs. 42% of generalists. To be honest since the number of questions that determine if you’re susceptible or critical is 2, I have to take this with a grain of salt.
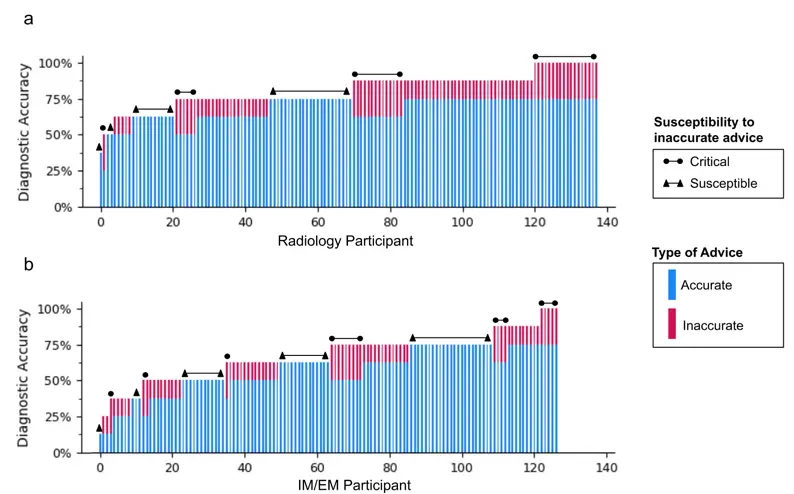
All in all it’s an interesting paper that surfaces important conclusions around how physicians anchor to advice. The discussion section has an interesting point around how you can actually have back-and-forths when asking colleagues for advice, which helps understand the level of confidence that the advice giver has. Being able to show the level of confidence in support tools is important to combat a lot of the inherent biases we already have.
In addition, unlike getting advice from another person, automated recommendations typically do not provide an opportunity for the back-and-forth conversations that characterize many physician interactions, nor involve any notions of uncertainty. Previous research has shown that people prefer advice indicating uncertainty and are more likely to follow sensible advice when provided with notions of confidence37. Developing tools that accurately calculate measures of confidence and display them in an understandable way is an important research direction, especially if they may be used by physicians with less task expertise who are at a greater risk to over-trust advice. Indeed, in our study, we found that while physicians often relied on inaccurate advice, they felt less confident about it. Tools that can understandably communicate their own confidence and limitations have the potential to intervene here and prevent overreliance for these cases where physicians already have some doubt
It’s a good paper, I recommend reading it. I kind of wish they had a couple questions where NO advice was given at all to provide a baseline, but still answers a lot of important questions.
U-S-A, U-S-A, E-H-R, U-S-A
Two questions I ask myself every day.
- Where is Bobby Shmurda’s hat?
- Are electronic health records (EHRs) uniquely bad in the US or everywhere
Here’s a cool paper that looked at where physicians in different countries spend time in Epic, one of the largest EHR systems. Each Epic build is a unique special snowflake that generally reflects the needs of a given system (specifically - their billing needs and associated documentation for that billing).

*As a caveat, the paper looked at 348 US health systems and 23 non-US systems (of which 10 were in the Netherlands).*
The way they did this was cool - they looked at the metadata of how people were interacting with the EHR. I don’t want to know who they have hostage to be able to get access to this data. But it can log things like keystrokes, mouse movements, scrolling, etc. in some twisted, “Strava for watching your computer” sick fantasy.
The software has the capacity to monitor EHR activity at an extremely granular level, collecting metadata primarily with the Signal data extraction tool. Those metadata document the time the system is used, as indicated by keystrokes, mouse movements, clicks, scrolling, and interactions with the EHR. In this analysis, time was defined as the time a user was performing active tasks in the EHR. If no activity is detected for 5 seconds, the system stops counting time. This time measure captures active EHR engagement and excludes other time a clinician spends performing nonkeystroke tasks while the EHR is open.
That data is useful to see how much time is spent in different modules. Lots of interesting findings but a few things jumped out at me.
- Docs in the US spend 90 minutes per day in the EHR vs. 58.3 minutes in other countries. Some are spending 2.5 hours+, pour one out for them.

- In the US, the notes are longer but also much more automated as a percentage of the note text. A lot of dot phrases just flying around everywhere.
- In the US, much more time is spent messaging (~34 per day for US physicians vs. ~13 for non-US). Anecdotally, physicians I’ve talked to have pointed to how many messages they get from patients through the portal, system messages bugging them to do things, and messages flying around from other team members. I’m sure this contributes to the enormous alert fatigue physicians have.
It also makes me wonder if this is something that can be improved via better collaboration tools, communication triaging (e.g. chatbots or other clinical staff answering patient questions, etc.) or if it’s just that these conversations happen in the EHR in the US vs. off the EHR in other countries and it’s just a part of medicine.

- Physicians at community hospitals and safety net providers spent 10 and 15 minutes more per day respectively in their EHRs compared to their Academic Medical Center counterparts. This may not seem like a lot, but in areas where physicians are particularly overstretched this makes a difference.
- One existential question about this study that Brendan Keeler pointed out is how much of this is just due to the EHR system in the US being more mature? We have a well built out ePrescription network thanks to SureScripts which probably explains why more prescription related stuff happens in the US EHR. There are also more hospitals with other Epic systems so cross hospital messaging would increase.
One of the meta-reasons I also like this paper is because after tweeting it there was a significant discussion with the author of the paper itself, who even ran some ad-hoc analyses. I wish each paper had sidebar annotation-style commenting like Google Doc comments where you had more social peer-review like this. It feels like there are so many good discussions happening about different published papers that get scattered everywhere without everyone else able to see it.
Anyway, lots of great additive discussion from the author here that was helpful in contextualizing this paper for me. His point about generally over-documenting everything because you’re not sure which payer you have to deal with and while they’ll deny something feels correct.

If You Need Me, Call Me, No Matter Where You Are
I like this study because the intervention itself was really simple.

Take 16 college age people, give them a one hour seminar on how to have an empathetic phone call, and have them call elderly Meals on Wheels recipients over the course of 4 weeks. See how the depression scores of the call recipients change over time vs. a control group that received no calls. That phone call empathy course would probably be useful for some of the investors I know 🙃.

It does seem like in general the group that got the phone calls seemed to improve using most scales measuring depression and other behavioral health assessments. This is one of those interventions that just makes logical sense - people will feel better if you call them at a time when people are at their most lonely. This study is great because it doesn’t over complicate it - no CBT techniques, asynchronous chatbots, etc. Just a 15-20 minute phone call every so often. And it’s cheap - the college students were volunteers given a $200 stipend and each had a panel of 6-9 participants.

Could the study have tracked this over a longer period and seen if those mental/behavioral health improvements led to physical ones? Sure, but that would have been longer and lots of other literature has already drawn the conclusion between depression and health outcomes in older adults. This experiment kept it short and directly measurable (guessing there are less confounding variables during COVID, and COVID should have made things worse mentally if anything).
To me the study showed we can improve the lives of the elderly without needing to medicalize the process with certifications or fancy techniques. And we can do that with people less trained - like college students. The Papa team must be absolutely giddy seeing a study like this.
I think it would have been interesting to see if the CALLERS actually saw their mental/behavioral health improve at the end of the experiment. A survey of ~33K college students in fall 2020 saw 60% screen positive for depression, a fifth were considered major. What if calling seniors had actually improved the PHQ-9 scores of the callers as well?

One last thing about this study is that it piqued my interest around the scales themselves. The two most prominent scales to measure loneliness seem to be the De Jong Giervald Loneliness Scale (De Jong) and the UCLA Loneliness Scale. The AKON loneliness scale never quite took off.
But between these two scales, this experiment saw an improvement in the UCLA but not the De Jong Scale. Actually it turns out that this is a long running debate with lots of history - the UCLA scale was mostly tested on younger adults and the two seem to have different beliefs on how loneliness manifests. This paper makes the argument that the De Jong scale is more useful when evaluating older adults that look at depression more “cognitively” than just socially. If that scale is more useful maybe this intervention didn’t actually help that much?
What it actually signals to me is that the scales themselves need to be updated, they’re both 15+ years old and require filling out surveys with questions that are trying not to be too loaded but clearly are kind of loaded. I feel like this is a great area for digital biomarkers to thrive - can monitoring your phone activity actually give a more passive and possibly objective capture of depression? There are DEFINITE questions around privacy there, but just an open thought on the need for new measures here.
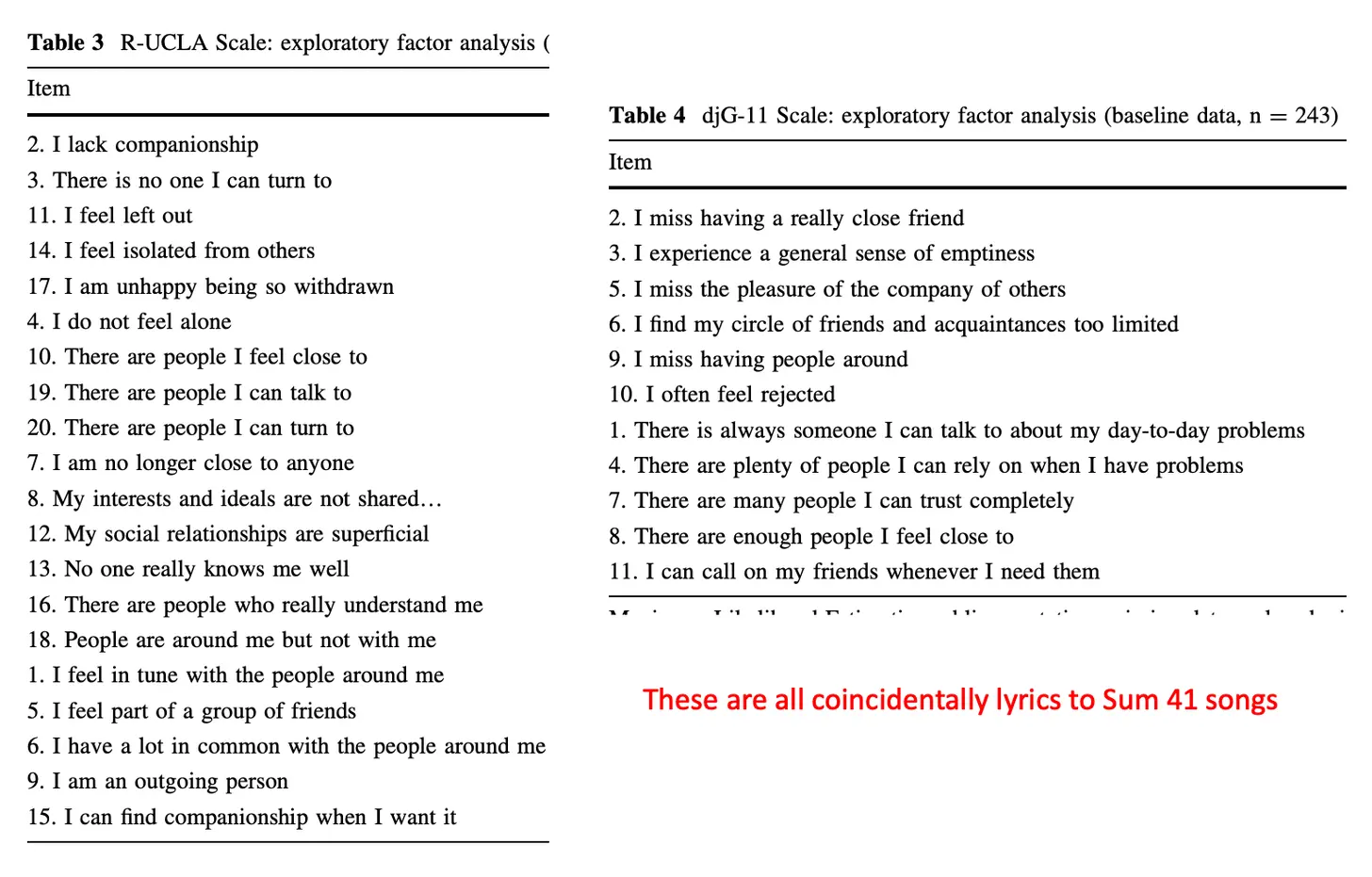
All in all, simple study with a simple cost-effective intervention. Good stuff.
Thinkboi out,
Nikhil aka. “Dr. Robot-Nik”
Twitter: @nikillinit
Interlude - Courses!!!
See All Courses →We have many courses currently enrolling. As always, hit us up for group deals or custom stuff or just to talk cause we’re all lonely on this big blue planet.