How Data Transformed Small Group Underwriting
Get Out-Of-Pocket in your email
Looking to hire the best talent in healthcare? Check out the OOP Talent Collective - where vetted candidates are looking for their next gig. Learn more here or check it out yourself.
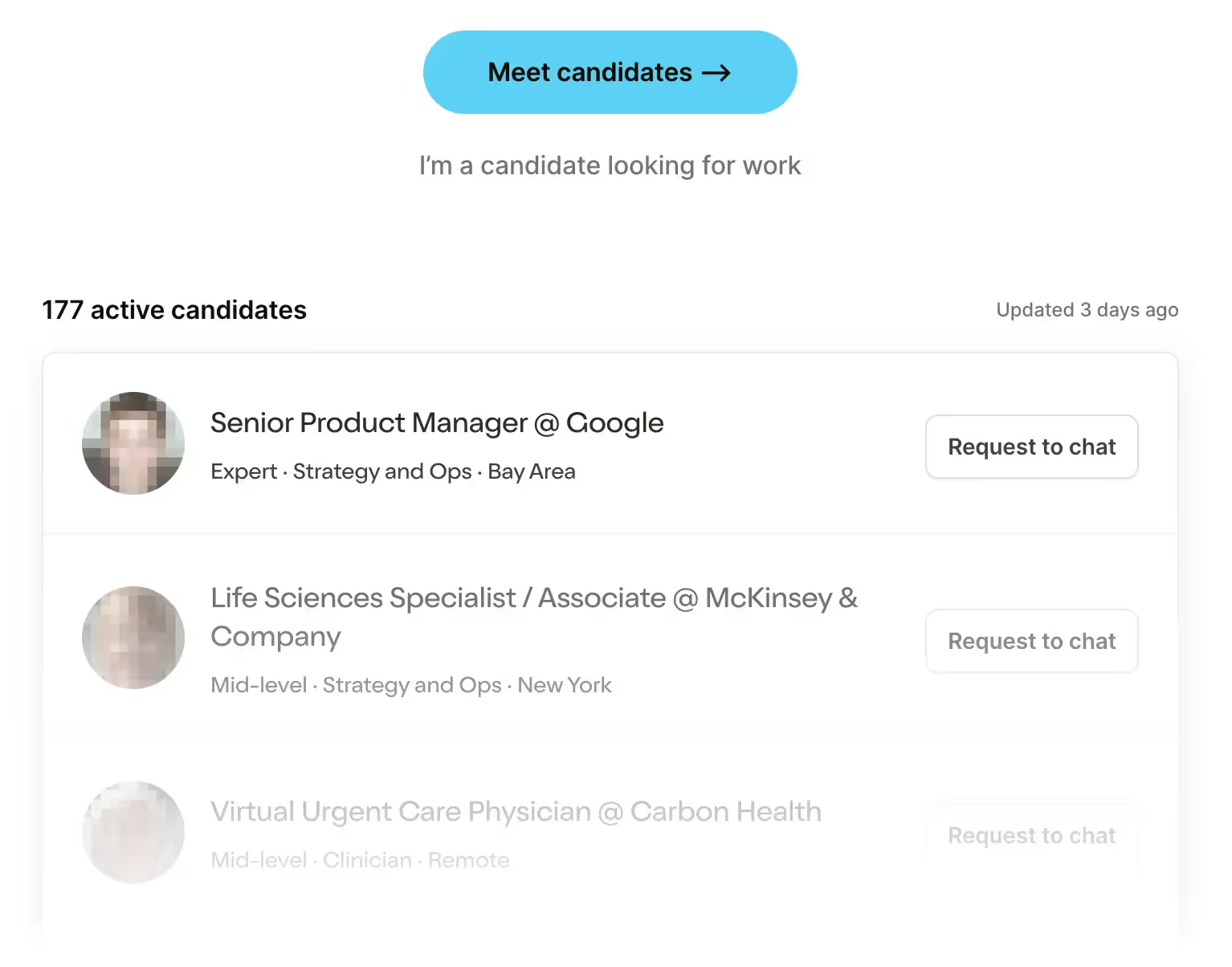
EHR Data 101
.gif)
Featured Jobs
Finance Associate - Spark Advisors
- Spark Advisors helps seniors enroll in Medicare and understand their benefits by monitoring coverage, figuring out the right benefits, and deal with insurance issues. They're hiring a finance associate.
- firsthand is building technology and services to dramatically change the lives of those with serious mental illness who have fallen through the gaps in the safety net. They are hiring a data engineer to build first of its kind infrastructure to empower their peer-led care team.
- J2 Health brings together best in class data and purpose built software to enable healthcare organizations to optimize provider network performance. They're hiring a data scientist.
Looking for a job in health tech? Check out the other awesome healthcare jobs on the job board + give your preferences to get alerted to new postings.
Health Insurance - Big Problem For Small Business
You thought we were niche before? Oh, I’ll show you niche.
I’ve been going down the rabbit hole of insurance for small businesses. This has been motivated by a few things.
- I’m lonely.
- I hang out with a lot of startup founders who complain to me about their insurance and then expect me to produce some “hack” that lowers their insurance costs by 50%.
- Everyone I talk to says the small group insurance market is in a bad place but I don’t really know why and that bugs me.
In general, I’m in my “small businesses are the backbone of America” era. A classic. I want to understand how we make it easier to start a small business in the US. One of the biggest impediments is cost of labor, of which health insurance is an extremely fast growing part of that.
So I’m going to be writing a few posts that explain different parts of this slice of health insurance. Today, I want to start with how underwriting works because it’s a key part in understanding everything else.
Small Group Health Insurance, Quick Primer
IT’S DEFINITION AND CONTEXT SETTING TIME OH BOY OH BOY.
Small businesses typically are “fully insured”, which means they aren’t taking on the financial risk of their employees. They pay health insurance companies premiums, and the health insurance companies take on the financial risk. If one employee gets really sick, the health insurer ends up paying for it. If a business were “self-insured”, they’d be picking up that employee’s bill themselves.
Small businesses that are fully insured are typically thrown into something called “small group” insurance. This is a “small group” in the way most Indian families consider something a “small group”...50 people. This counts as groups of <50 employees in most states and <100 employees in a handful of states (New York, California, Colorado, Vermont). ~8.5-9M people are covered in the small group market, and ~16M including dependents.
Depending on the state, employers do not legally have to offer health insurance to their employees if they’re <50 employees. Some do so in order to stay competitive in the labor market. Others do it out of the goodness of their hahahahaha. And yet, the number of small employers offering health insurance over time is decreasing. It’ll make sense why by the end of the post.
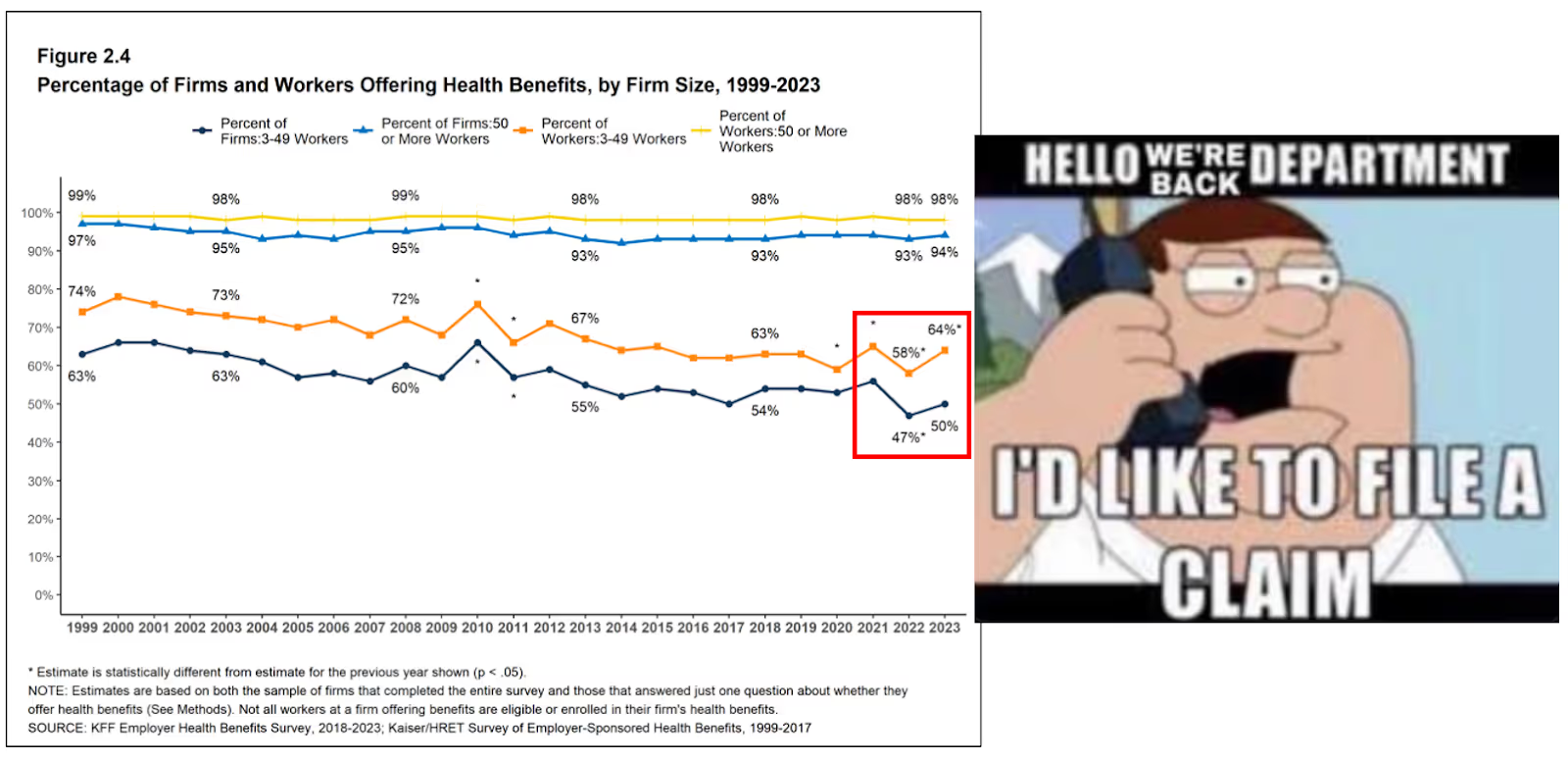
There are some big differences between the small and large group markets, but the biggest one has to do with how insurance is underwritten between the two groups. This is what I want to talk about today.
How Underwriting Works at a High Level
For simplicity's sake, let’s go over two types of underwriting we need to understand. I’ve already lost half of you at this point, so I need to keep it simple for the survivors.
Modified Community Rating
Small groups use “modified community rating” which allows you to change premiums per person for a few criteria.
The characteristics you can underwrite on are pretty limited. All of them are related to characteristics of the person and not their health status (like age, gender, smoking status, etc.). Each state allows some different permutation of these factors in their underwriting, which you can see here.

These characteristics usually have a “band ratio”. This limits how much you can charge the most expensive person. For example, a 3:1 age band ratio means a 64 year old can only be charged 3x more than a 21 year old. This is tricky because the 64 year old is more than 3x as expensive as a 21 year old - so the rate you charge 21 year olds needs to be higher than their actual risk to offset that.
You’ll see these band ratios across all those different characteristics above (e.g. 1.5:1 smoking status ratio, 2:1 incel ratio).
Using this underwriting methodology is mandatory due to the Affordable Care Act if you’re selling to small groups.
Experience Rating
If you’re a large group or self-funded plan, you’re underwritten using “experience rating”. In this type of underwriting, insurers actually look at how the group has used the healthcare system and adjust premiums accordingly. What was the group’s spend the last few years? What kinds of conditions might they have? Why did two people have an ICD code of S10.87XA on the same day? What types of medications are they on? Etc.
—

As you might imagine, community rating is tougher to accurately price premiums. You have fewer factors you can use to assess the health of patients and you’re limited in how much more you can charge the riskier patients.
Experience rating uses historical healthcare usage data to predict future costs. Thanks to AI, better underwriting, a blind eye to privacy, and more data, we can get an even more precise picture of what costs look like.
The delta in precision between these two is increasing. Pricing insurance based on expected risk is effectively how all other insurance markets (auto, life, etc.) price their products. But the Affordable Care Act wanted to put provisions that prevented different types of discrimination, including limiting the ability for insurers to underwrite on pre-existing conditions and denying insurance coverage to small groups.
If you’re a small group with sicker employees, community rating is great because you’ll be mixed with all the other small groups with healthy employees and therefore get better rates. But if you’re a small group with healthy employees, you might feel that you could’ve gotten better rates if they’d only underwritten your group alone. I feel like everyone reading is looking at their coworkers now; please stop doing that.
These Affordable Care Act rules are well-intentioned, but only work if all the insurance companies are playing by the same rules. If you were a betting person…do you think they all do?
A New Era of Data-Driven Underwriting
One of the main issues in pricing premiums accurately for experience rating has been actually getting data from patients to do this underwriting appropriately.
How do you get data to accurately predict risk if you’re allowed to? In the past, most small businesses that have either never had insurance before or are fully insured would have to ask their employees for this information. One such form employers could have employees fill out is called an IHQ (Individual Health Questionnaire). Do you remember this clip from The Office? These forms are annoying to process and awkward to collect - giving my boss a form that says I have burning pee pee seems bad.
However, this has changed in the last decade thanks to tokenization. We did a primer of how this works a few years ago, but the general gist is that data brokers can essentially de-identify a patient in individual datasets but know it’s the same patient across different datasets. This allows companies to link datasets together. You don’t know it’s “John Smith”, but “patient 1125089” is the same patient across medical claims and pharmacy claims so you can join them.
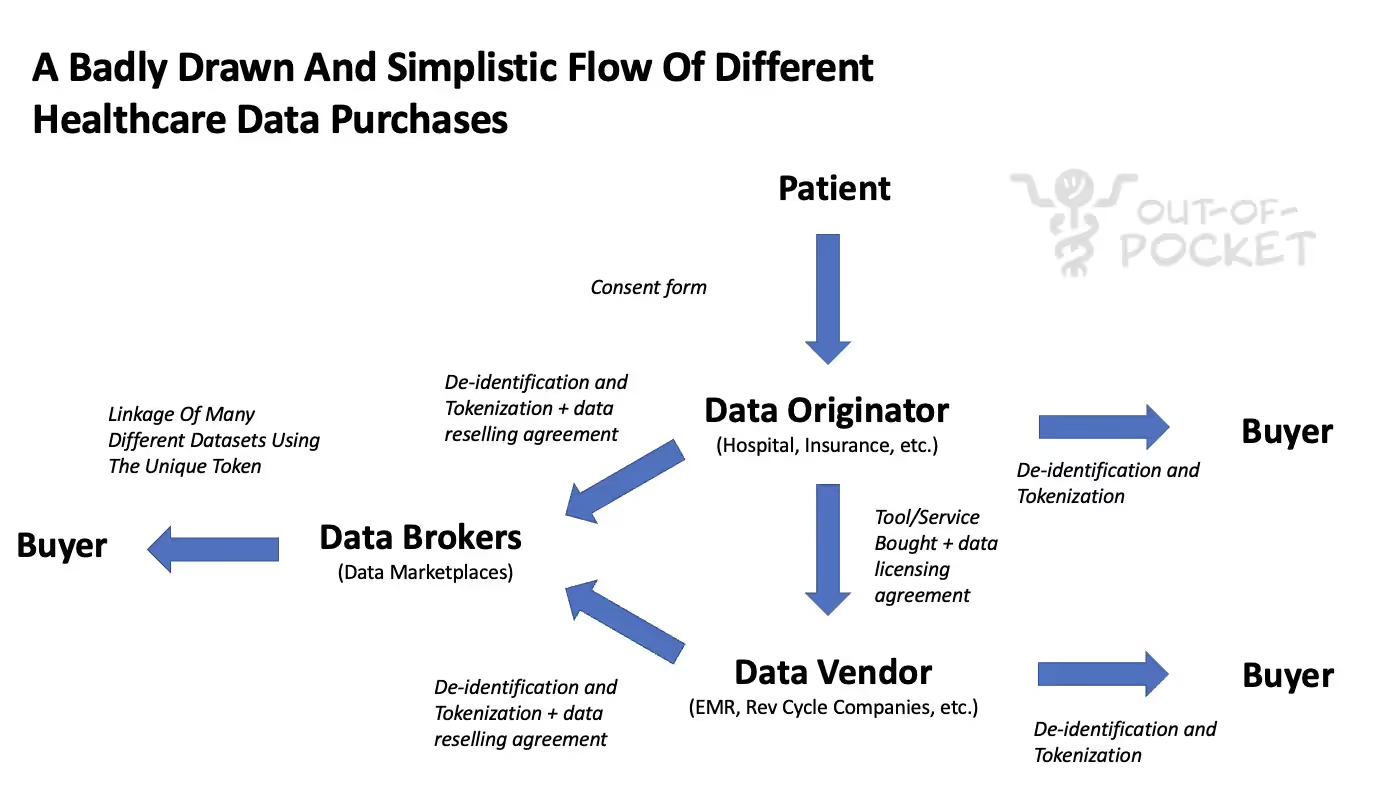
That same tokenization technology can now much more easily underwrite the risk of groups of employees. An employer basically gives a census file of their employees and dependents to one of these more sophisticated underwriting vendors. That census file contains a lot of standard info (name, date of birth, gender, zip code, etc.) that you’d likely have to collect to run payroll, though dependents’ information might require some hunting down.

Using that census file, these more sophisticated underwriters like Milliman Curv, Gradient AI and Verikai can take a group of employees and link it to various healthcare datasets. The most common linkage is claims data, which the clearinghouses sell de-identified and en masse. But it’s getting even wider than that - in this podcast they talk about how AI now allows brokers to underwrite groups combining pharmacy data, driving records, social media, etc. to generate a much more specific risk score.
The key here is that you’re getting the risk of an entire group via a de-identified means, so you don’t actually need individual consents. Just another example of businesses having fun with our data when individuals struggle to do the same linkage. Hip, hip, HIPAA!
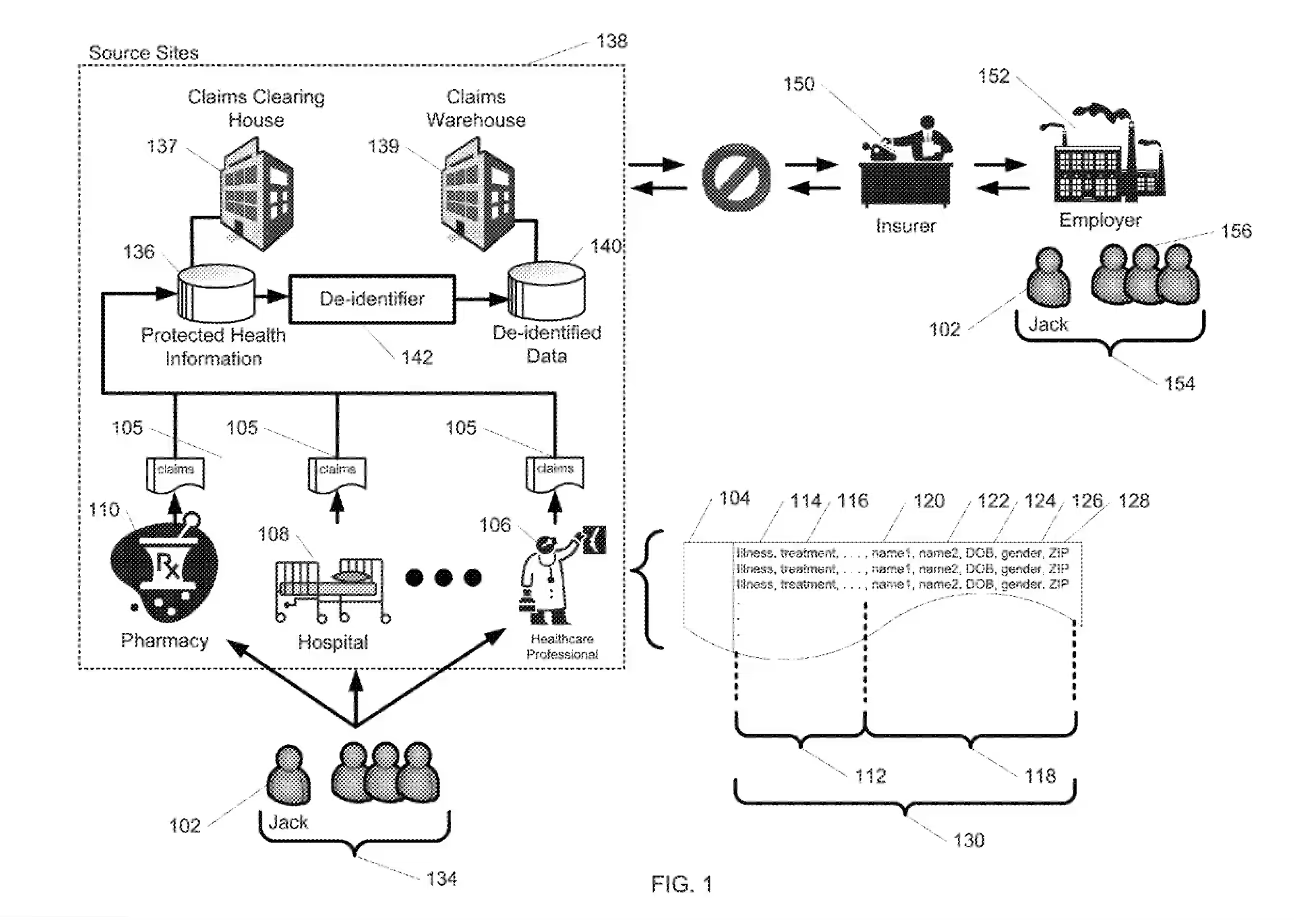
This data can give you a general sense of what kind of spend and risk a group of employees represents. But still, it often has gaps and issues. Especially when you’re trying to figure out how much the really expensive patients actually cost, since their data lives in a lot of different places.
However this is already a big unlock for three reasons:
- Brokers can use tools like this to quickly figure out if small businesses can get better rates by exploring other options like some form of self funding.
- The lift for carriers that might want to quote to smaller employers doesn’t require going to employees one by one.
- The process is MUCH faster, which is important since there’s a small time window every year when employers pick their health plans.
Intermission - The EHR Data Course
Did the paragraph about tokenization make you a little data promiscuous? Interested in learning about how to do analysis with electronic health record data?
Fear not, our EHR data 101 course is out. You’ll learn everything about how EHR data is structured, things to watch out for if you’re analyzing this kind of data, and some real-world examples of how to use it for different use cases.
You can sign up here and see the full curriculum. Email philip@outofpocket.health for group discounts.

[Oh also, we just opened up enrollment for our How to Sell to Health Systems course this September. You can see more details about that here.]
The Evolution of Stop Loss Insurance
At the beginning of this we talked about self-funding, which is when a company takes on the financial risk of their employees. If an employee gets a medication that’s $200K a year, the employer is now paying for that themselves. As you can imagine, if you’re a small company, you can’t afford to take on this risk since it could potentially bankrupt your company.
At a certain point, a company becomes large enough that it feels like it can absorb the costs if someone gets sick. But most companies still get a type of insurance that protects against really crazy bills - like if one person costs $1M dollars or something like COVID where a bunch of people generate a ton of $100K bills at the same time. This is called “stop loss insurance”, a name so boring and literal it must have been named by an actuary.
Stop loss insurance plays a pivotal role here. An employer usually will not take on the medical costs of their employees without a stop loss carrier unless they like rawdogging risk. For the stop loss carrier, everything relies on the underwriting of those employees.
[It’s worth noting that stop loss carriers are usually companies that are just giant pools of money, and they aren’t specific to healthcare. Sun Life, AllState, etc. have lots of different insurance and financial products like life insurance, car insurance, etc. These carriers typically rely on third party Managing General Underwriters (MGU) who are more specific to underwriting healthcare risks.]

Tokenization makes it possible to quickly but less specifically assess group risk. But stop loss needs to get much more data on specific patient risk when it comes to the high cost employees that they’ll be on the hook for. Data has changed stop loss in many ways, but there are a couple big ones I want to highlight.
- Enhanced health questionnaires- Remember when we said before that employees would have to fill out questionnaires to understand group risk? Nowadays, a group might get a quick snapshot of risk using the de-identified risk scoring stuff we talked about before. But if it seems like a few people are really expensive, some stop loss carriers will want to know more about them.
Today several products make it easier for employees to aggregate their data into one place and send it vs. trying to remember to fill the forms out. For example, this company Rover seems to have employees give their login credentials to each payer and then pulls their data. This lets the stop loss carrier get a better sense of the kind of costs they’re dealing with. - Lasering - One version of stop loss insurance basically says if any person goes above $100K, the stop loss carrier will cover above that. But when someone is expensive, they’re usually REALLY expensive. So sometimes a stop loss carrier will create a “laser”, a name so cool an actuary definitely didn’t name it. While the employer covers the bill up to $100K for everyone else, for this one employee in particular they have to cover the bill up to $250K. By creating these exceptions for high cost employees where the employer eats more of the cost, the stop loss carrier is more willing to underwrite the group as a whole.

- Proactive care management. This is more of an emerging area. With more data about employees, stop loss carriers can identify both already high cost patients but also rising risk patients. This also means that brokers can work to combine stop loss carriers with benefits/advisors that might help patients that are particularly high cost. For example, they may switch them to a cheaper drug or hospital using care navigators. A carrier is going to be more willing to underwrite a group if it knows more specifics around what’s driving the high costs and whether the employer/broker has ways to manage those costs.

So why does this all matter? With access to more data and analysis like this, stop loss carriers are more willing to underwrite increasingly smaller groups of employees. This has opened the door to more arrangements where small businesses take on the financial risk of employees themselves if they feel like their employees are particularly healthy without needing to worry about going bankrupt.
Conclusion - The Fracturing of Small Group Insurance
The key point I want to get across here is that the risk level of any small group is much more visible.
An insurance underwriter can more easily figure out which groups are healthier vs. sicker using a handful of data points and without the need for patient consents. Brokers and benefits consultants can go to small businesses and tell them they have healthier employees so they’re overpaying for their current plan, since that plan has to adhere to modified community rating rules.
But then what happens? The small group with healthy employees leaves the traditional small group people. The only people left in the traditional pool are employers with particularly sick groups of employees. And what happens to their healthcare costs? They go way up. This is one of the reasons why the remaining small groups offer plans with higher deductibles, more restrictive provider networks, or no health coverage at all.

In follow up posts we’ll talk about the kinds of plans small groups are changing to, specifically in areas like Professional Employer Organizations (PEOs), ICHRAs, level-funding, and more. We’ll also talk about how self-insured plans are regulated totally differently.
But we need to have a baseline understanding of how the underwriting itself has changed before we go further.
**A Side Note On Privacy And Underwriting
It’s also worth flagging that there are a lot of questions about patient privacy when it comes to underwriting. Many of those entities aren’t governed by HIPAA, which many of these vendors lay out in their terms of service.
On top of that - HIPAA doesn’t protect the privacy of a group of individuals, just individuals. I can own deidentified statistics about groups of people. A lot of these underwriting tools are just doing “statistical correlations” on groups of people.

When you get into increasingly small groups of people, it doesn’t take much to start getting into gray areas around whether a dataset is really deidentified. I’m also pretty certain that most employees at companies don’t understand that their data gets used in this way. Company CEOs I’ve talked to that have gone through this have felt “the ick”.
Then again, do you understand how your data gets used anywhere or do you just say “accept all cookies” on the site?
Thinkboi out,
Nikhil aka. “The smallest possible group” aka. “Band Ratioz A Make her Dance”
Thanks to Russell Pekala and Alan Vandagriff who reviewed drafts of this.
Twitter: @nikillinit
IG: @outofpockethealth
Other posts: outofpocket.health/posts
--
{{sub-form}}
If you’re enjoying the newsletter, do me a solid and shoot this over to a friend or healthcare slack channel and tell them to sign up. The line between unemployment and founder of a startup is traction and whether your parents believe you have a job.